Predicting Health Outcomes in Skilled Nursing Facilities
How do you identify a great skilled nursing facility? Is it possible to predict health outcomes in these facilities? This project looked at data on over 15,000 nursing homes in the United States in the hopes of answering those two questions.
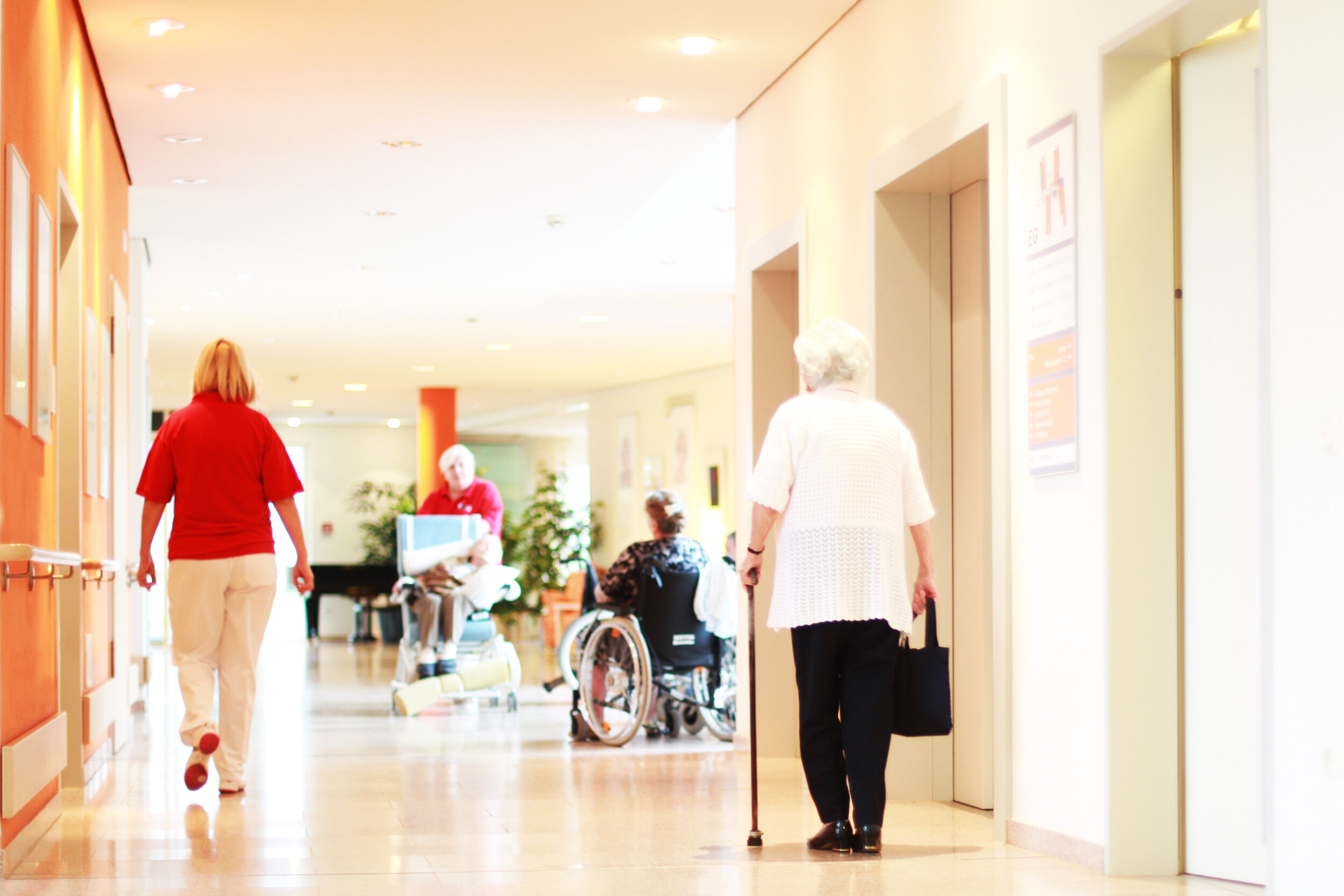
The Purpose
Over the last few years, while the COVID-19 pandemic shook the world, nursing homes were hit especially hard. The elderly population as a whole was already at a high-risk with the illness, but certain nursing homes fell flat in their prevention of spread and care for residents. In November of 2020, the Centers for Medicare and Medicaid Services handed out almost $18 million in fines to nursing homes for not providing adequate safety to residents during the pandemic.
Unfortunately, neglect and mistreatment of individuals in Skilled Nursing Facilities is not a new issue caused by the pandemic. The Nursing Home Abuse Center states that 1 in 10 of individuals over the age of 65 have experienced some type of elder abuse. All of this made me want to take a closer look at how the treatment and health outcomes of elderly in Skilled Nursing Facilities is tracked and monitored. The Center for Medicare Services has been tracking and reporting data from skilled nursing facilities across the country and my goal was to see if I could spot trends within their data around health outcomes.
The Process
I collected the 2021 CMS data for this analysis from their website. While the CMS does have an extensive network of APIs, I chose to bulk download the data I needed since the files are static and unchanging once uploaded. I then cleaned and combined the various files into a single file.
I engineered many different features out of the data. The ownership file originally had every individual or organization's name listed with their role. I thought ownership information could be a significant predictor of skilled nursing facilities’ performances I spent some time coercing out extra features.
The newly engineered features included:
Number of Individual and Organizational Owners: How many unique owners are listed for that specific facility?
Max and Mean Facilities Owned: What is the max and average number of other facilities that owners are listed as having an ownership stake in?
Max Roles: How many roles does the owner with the most roles have for that one facility? (example: some owners are listed as directors, officers and owners)
Owners with Same Last Name: Are there any owners of the facility with the same last name?
Individual & Org Owned: Are there both LLCs and individual owners listed?
Original Data:
After feature engineering:
I performed similar levels of engineering on the provider information and facility outcomes data files before merging them. To see all changes, refer to the notebook on GitHub here.
Exploratory Analysis
When analyzing the health outcomes of these facilities, I could have chosen several metrics reported by CMS, including:
Percentage of long-stay residents whose need for help with daily activities has increased
Percentage of long-stay residents who lose too much weight
Percentage of long-stay residents who have depressive symptoms
Percentage of high-risk long-stay residents with pressure ulcers
I chose not to use the more general measures like the percent of residents whose need for daily activities has increased or the percent of residents whose ability to independently walk has decreased. Many individuals enter Skilled Nursing Facilities because their health is declining, and they need more help. My intuition was that the outliers in those measures could offer a lot of insight into poor-performing facilities, but that because they are also a bit vague in their definition, it might be difficult to model.
Ultimately, I chose to use the percentage of long-stay residents with pressure ulcers for my model. Pressure ulcers are sometimes unavoidable because of illnesses, even with the best care. However, they are also often listed as indicators of neglect in nursing homes.
Across the United States, the range of reported percent of pressure ulcers starts at 0.5% and goes up to 59%. There are some clear outliers in the data since the average percentage is 8.4%, with a 4.8 standard deviation.
I looked at whether location had a big influence on the percentages reported and found that the state with the lowest percentages were:
Hawaii at 5.0%
Maine at 5.9%
Nebraska at 6.1%
The locations with the highest percentages were:
Washington, D.C. at 12.3%
Missouri at 10.9%
Nevada at 10.7%
There were not any strong correlations in any of the variables that I compared. Some of the staffing metrics had mild positive correlations. For example, RN hours per resident had a .20 correlation and LPN hours per resident had a .17 correlation. This makes sense as you would expect to see better patient outcomes with more staffing. The facility’s overall rating had a slightly negative correlation of -0.13 which would also be expected. I had hoped ownership information would be predictive but that proved to not have much of an impact.
When I removed looked just at facilities in the 75th percentile and above, they had a much stronger correlation to staffing hours and to a few of the other health outcome measurements like the percentage of residents who have lost too much weight.
The Model
From this point, I moved on to create a regression model. I split the data into testing and training sets and created a baseline to beat by predicting that every facility had the mean value of the dataset. From there, I tested several different models and optimized the parameters of each one using GridSearch. I used Mean Absolute Error to measure the success of each model.
Discussion
While Ridge Regression, Gradient Boosting, and Random Forrest had very similar metrics, Random Forrest did the best job of correctly estimating the outlier data. This isn't necessarily a surprise, given that Random Forrest and Decision Trees are better at dealing with nonlinear data than regression models like linear and ridge regression. It's also not surprising that Ridge Regression got many outliers incorrect since the algorithm is designed to minimize the impact that outliers have on its predictions. Overall, the models performed relatively well and beat the baseline.
In the future, I'd like to expand this model by creating categories based on the quartile ranges and see if, by using logistic regression methods, I can better predict whether or not a facility will perform below average. I have the intuition that this approach may be even more accurate since the Random Forrest Regression model worked the best.
In addition, I'd also like to include more data on the demographics surrounding the location of each Skilled Nursing Facility and take this data analysis back farther in time to see if there were significant changes due to COVID-19.